数据整理
library(vegan)
## Loading required package: permute
## Loading required package: lattice
## This is vegan 2.6-4
data("varespec")
pca <- rda(varespec)
首先看一下结果:
summary(pca)
##
## Call:
## rda(X = varespec)
##
## Partitioning of variance:
## Inertia Proportion
## Total 1826 1
## Unconstrained 1826 1
##
## Eigenvalues, and their contribution to the variance
##
## Importance of components:
## PC1 PC2 PC3 PC4 PC5 PC6
## Eigenvalue 982.9788 464.3040 132.25052 73.9337 48.41829 37.00937
## Proportion Explained 0.5384 0.2543 0.07244 0.0405 0.02652 0.02027
## Cumulative Proportion 0.5384 0.7927 0.86519 0.9057 0.93220 0.95247
## PC7 PC8 PC9 PC10 PC11 PC12
## Eigenvalue 25.72624 19.70557 12.274191 10.435361 9.350783 2.798400
## Proportion Explained 0.01409 0.01079 0.006723 0.005716 0.005122 0.001533
## Cumulative Proportion 0.96657 0.97736 0.984083 0.989799 0.994921 0.996454
## PC13 PC14 PC15 PC16 PC17
## Eigenvalue 2.327555 1.3917180 1.2057303 0.8147513 0.3312842
## Proportion Explained 0.001275 0.0007623 0.0006604 0.0004463 0.0001815
## Cumulative Proportion 0.997729 0.9984910 0.9991515 0.9995977 0.9997792
## PC18 PC19 PC20 PC21 PC22
## Eigenvalue 0.1866564 1.065e-01 6.362e-02 2.521e-02 1.652e-02
## Proportion Explained 0.0001022 5.835e-05 3.485e-05 1.381e-05 9.048e-06
## Cumulative Proportion 0.9998814 9.999e-01 1.000e+00 1.000e+00 1.000e+00
## PC23
## Eigenvalue 4.590e-03
## Proportion Explained 2.514e-06
## Cumulative Proportion 1.000e+00
##
## Scaling 2 for species and site scores
## * Species are scaled proportional to eigenvalues
## * Sites are unscaled: weighted dispersion equal on all dimensions
## * General scaling constant of scores: 14.31485
##
##
## Species scores
##
## PC1 PC2 PC3 PC4 PC5 PC6
## Callvulg -1.470e-01 0.3483315 -2.506e-01 0.6029965 0.3961208 -4.608e-01
## Empenigr 1.645e-01 -0.3731965 -5.543e-01 -0.7565217 -0.7432702 -3.484e-01
## Rhodtome -6.787e-02 -0.0810708 -6.464e-02 -0.0963929 -0.1749038 -4.008e-02
## Vaccmyrt -5.429e-01 -0.7446016 1.973e-01 -0.2791788 -0.5688937 -3.689e-01
## Vaccviti 9.013e-02 -0.7247759 -6.555e-01 -1.3366155 -0.7107105 -2.615e-01
## Pinusylv 3.404e-02 -0.0132527 4.465e-04 -0.0079399 -0.0012504 3.800e-03
## Descflex -7.359e-02 -0.0961364 6.485e-02 -0.0158203 -0.0741307 -5.328e-02
## Betupube -8.789e-04 -0.0008548 -6.637e-03 -0.0077726 -0.0072147 4.759e-04
## Vacculig -6.959e-02 0.2123669 6.410e-02 0.0136389 -0.0712061 -1.179e-01
## Diphcomp 3.807e-03 0.0333497 -1.026e-02 -0.0291455 -0.0086379 -2.263e-02
## Dicrsp -4.663e-01 -0.3675288 -1.143e-01 -0.2490898 0.7694280 1.225e+00
## Dicrfusc -1.176e+00 -0.4484656 -1.338e+00 2.1943059 -0.9187333 4.027e-02
## Dicrpoly -1.597e-02 -0.0326269 -6.610e-02 -0.1166483 -0.0369723 6.576e-02
## Hylosple -2.935e-01 -0.4217426 4.523e-01 -0.0656152 -0.0358715 -2.234e-01
## Pleuschr -3.890e+00 -4.3657447 2.343e+00 0.3747564 0.1476496 -3.060e-01
## Polypili -5.032e-04 0.0069583 7.660e-03 -0.0005528 -0.0034856 5.849e-03
## Polyjuni -9.888e-02 -0.0584117 -7.234e-02 -0.0518007 0.0438920 1.472e-01
## Polycomm -4.028e-03 -0.0057429 -5.248e-03 -0.0104641 -0.0058180 9.059e-05
## Pohlnuta 9.473e-03 -0.0116498 -1.010e-02 -0.0112498 -0.0009520 7.691e-03
## Ptilcili -2.749e-02 -0.0257213 -2.522e-01 -0.3745203 -0.2917694 -4.907e-03
## Barbhatc -6.264e-03 -0.0070227 -7.152e-02 -0.1029116 -0.0825601 -1.183e-03
## Cladarbu -7.281e-01 3.0180666 2.016e-01 0.1400429 0.8708266 -1.253e+00
## Cladrang 8.117e-01 4.2257879 2.338e+00 0.2831533 -0.7644829 4.473e-01
## Cladstel 9.580e+00 -2.0074825 6.078e-01 0.4155714 0.1401899 -2.112e-01
## Cladunci -3.796e-01 0.0706264 -6.867e-01 0.1413783 0.9384995 1.495e-01
## Cladcocc 5.652e-03 0.0096766 -3.946e-03 0.0117038 0.0033077 -4.020e-03
## Cladcorn -1.367e-02 -0.0047214 -1.714e-02 -0.0270900 0.0004555 1.197e-03
## Cladgrac -1.037e-02 0.0083562 -6.489e-03 -0.0132067 0.0018124 1.012e-02
## Cladfimb 3.163e-03 0.0028937 -1.436e-02 0.0004203 -0.0043761 -1.344e-02
## Cladcris -2.649e-02 0.0010675 -4.626e-02 -0.0128097 0.0150875 -3.677e-02
## Cladchlo 1.347e-02 -0.0054141 -7.871e-03 -0.0099859 -0.0028496 -4.324e-04
## Cladbotr -2.051e-03 -0.0009378 -5.815e-03 -0.0095913 -0.0057131 -1.141e-03
## Cladamau 3.733e-05 0.0020956 3.925e-04 -0.0009025 -0.0009981 2.615e-04
## Cladsp 6.254e-03 -0.0021214 -1.997e-03 0.0011793 0.0023250 -3.246e-03
## Cetreric -4.938e-03 0.0079861 -1.740e-02 0.0046582 0.0488265 1.964e-02
## Cetrisla 1.715e-02 -0.0103936 -1.530e-02 -0.0214109 -0.0140577 3.490e-03
## Flavniva 8.793e-02 0.0932642 -3.761e-03 0.0536027 0.1450323 1.915e-03
## Nepharct -5.549e-02 -0.0368786 -3.638e-02 0.0055964 0.0415127 1.027e-01
## Stersp -5.531e-02 0.3478548 2.425e-01 0.0088566 -0.1772134 2.711e-01
## Peltapht -3.661e-03 -0.0014739 1.729e-03 -0.0067221 -0.0043042 -5.434e-05
## Icmaeric -1.435e-03 0.0040087 1.296e-03 0.0023703 -0.0024341 2.959e-03
## Cladcerv 8.746e-04 0.0001554 2.667e-05 0.0004662 0.0006877 7.336e-04
## Claddefo -5.274e-02 0.0023107 -8.009e-02 -0.0278485 0.0198955 -1.490e-02
## Cladphyl 1.581e-02 -0.0057716 5.064e-04 0.0010606 0.0027075 -1.441e-03
##
##
## Site scores (weighted sums of species scores)
##
## PC1 PC2 PC3 PC4 PC5 PC6
## 18 -1.02674 2.59169 -1.53869 -3.23113 -1.104731 -2.20341
## 15 -2.64700 -1.62646 1.01889 1.88048 1.152767 -1.41888
## 24 -2.44595 -2.01412 0.12182 -2.44215 5.875564 8.03640
## 27 -3.02575 -4.32492 4.44163 -1.54364 -2.466762 -2.57141
## 23 -1.86899 -0.35380 -1.98920 -4.11912 -2.428561 -0.85734
## 19 -0.02298 -1.59265 -0.43949 -1.43686 1.058122 -1.18048
## 22 -2.53975 -1.70578 -4.10001 7.63091 -5.062274 -0.64415
## 16 -2.08714 0.06163 -2.32296 5.84188 -3.568851 1.63566
## 28 -3.77083 -5.80241 6.57611 0.02751 0.908046 -3.10610
## 13 -0.38714 2.82852 -0.32352 2.12507 3.631355 -3.88730
## 14 -1.75570 0.75366 -5.69429 1.68697 6.098213 -1.28171
## 20 -1.65653 0.32765 -1.93100 -2.50536 0.065456 1.72887
## 25 -2.39601 -1.83554 -1.65364 0.24230 1.756334 4.36881
## 7 -1.08594 5.78134 1.89095 -0.90494 -0.006891 -2.44158
## 5 -0.80203 6.28624 4.85360 0.70195 -3.707269 5.90752
## 6 -0.19764 5.11581 1.24710 -0.70641 1.876853 -4.68229
## 3 3.79496 1.11636 1.93079 1.75008 -0.164043 1.75417
## 4 1.24779 1.77832 -0.17925 1.13363 2.999648 0.07212
## 2 5.49157 -0.67853 1.58337 1.13337 -1.472922 1.97780
## 9 6.02767 -3.10958 -1.09190 -0.28517 0.894284 -1.20958
## 12 4.19915 -1.40814 -0.06645 -0.79569 -0.790560 -0.37424
## 10 6.18267 -2.32211 -0.76545 0.43683 0.430813 -0.78609
## 11 1.09782 0.55023 3.41127 0.38027 -0.325506 1.07113
## 21 -0.32554 -0.41738 -4.97967 -7.00076 -5.649086 0.09209
绘图
vegan
的结果处理成 data.frame
才能用于 ggplot2
。这个过程比较复杂,不过还好有人已经造好了轮子:ggvegan
1。
运行下面命令安装这个包。
devtools::install_github("gavinsimpson/ggvegan")
使用 autoplot()
。
library(ggvegan)
## Loading required package: ggplot2
# 使用空白主题
theme_set(theme_bw())
# 一键绘图
autoplot(pca)
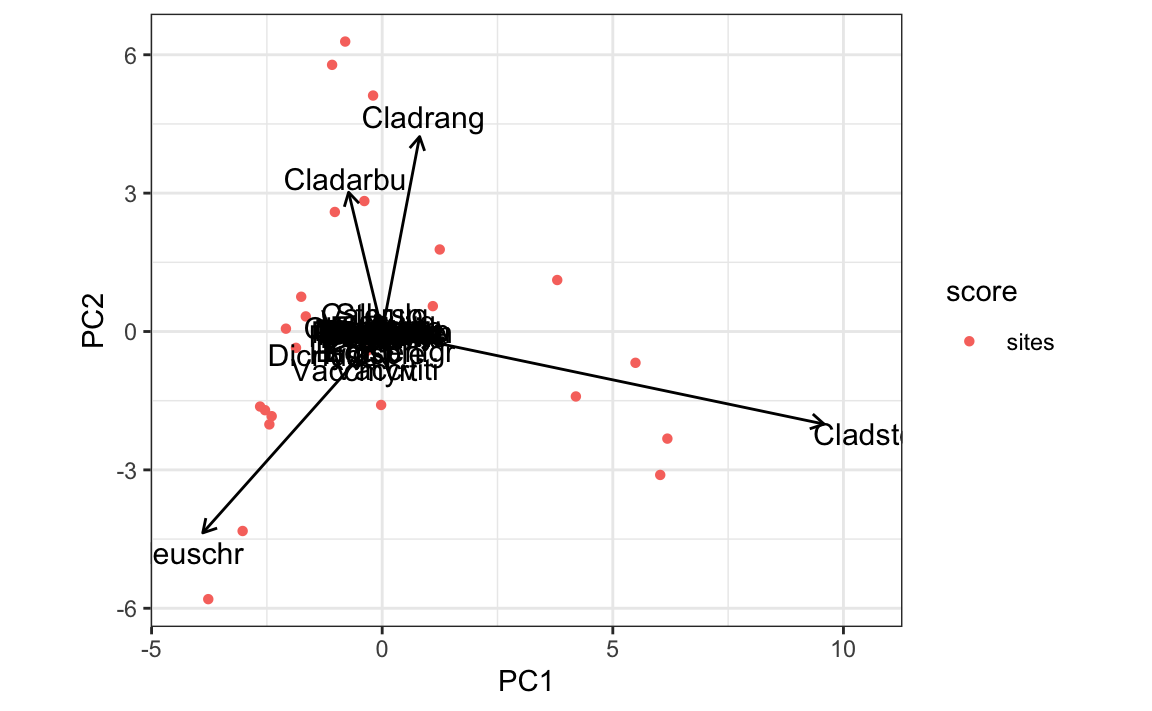
还可以使用 fortify()
将对象转变为 data.frame
,然后再作图。
# 生成数据框
df.pca <- fortify(pca)
# 查看数据
knitr::kable(df.pca[1:5,1:5])
score | label | PC1 | PC2 | PC3 |
---|---|---|---|---|
species | Callvulg | -0.1469634 | 0.3483315 | -0.2505840 |
species | Empenigr | 0.1645106 | -0.3731965 | -0.5543083 |
species | Rhodtome | -0.0678718 | -0.0810708 | -0.0646412 |
species | Vaccmyrt | -0.5428697 | -0.7446016 | 0.1973452 |
species | Vaccviti | 0.0901303 | -0.7247759 | -0.6554941 |
# 绘图
require(dplyr)
## Loading required package: dplyr
##
## Attaching package: 'dplyr'
## The following objects are masked from 'package:stats':
##
## filter, lag
## The following objects are masked from 'package:base':
##
## intersect, setdiff, setequal, union
ggplot(mapping=aes(PC1,PC2,shape=score,color=score)) +
geom_point(data=dplyr::filter(df.pca,score=="sites")) +
geom_segment(data=dplyr::filter(df.pca,score=="species"),
mapping = aes(x=0,y=0,xend=PC1,yend=PC2),
arrow = arrow(length = unit(0.1,"inches")),show.legend = F) +
geom_text(mapping = aes(PC1,PC2,label=label),data=filter(df.pca,score=="species"),show.legend = F)
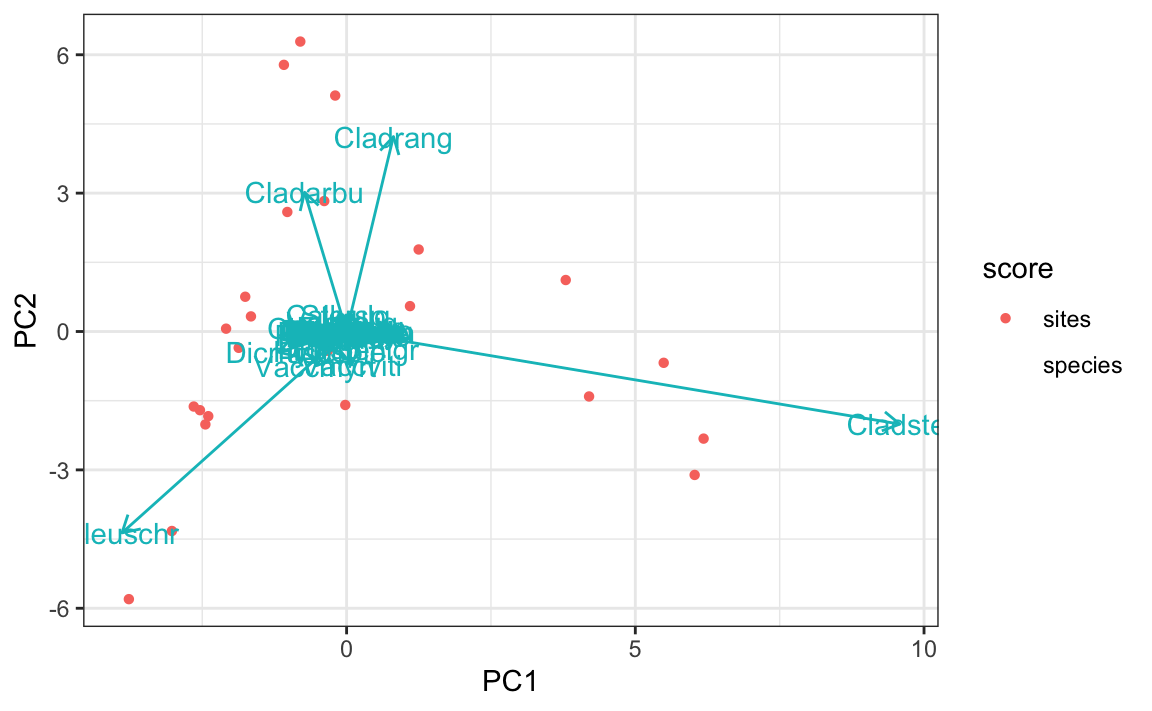
除了PCA分析,ggvegan
还顺便支持了CCA,RDA,metaMDS等vegan
的分析结果。
-
ggvegan on GitHub: https://github.com/gavinsimpson/ggvegan ↩︎